CEB Seminar - Identifying Heterogeneous Treatment Effects using Machine Learning as a Future Precision Medicine Approach - A/Prof Kosuke Inoue, MD, PhD, Department of Social Epidemiology, Kyoto University
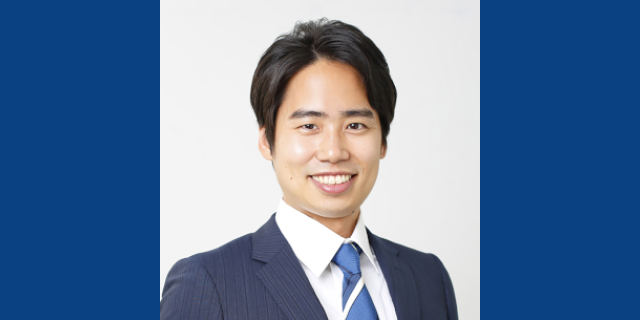
Kosuke Inoue
Abstract: In medicine, clinicians treat individuals with a high risk of diseases under an implicit assumption that risk is correlated with benefit. However, high-risk patients are not always the ones who benefit most from the treatment, and treating individuals with the highest estimated “benefit” (rather than “risk”) may improve population health outcomes. In this presentation, I will introduce a machine learning algorithm to assess heterogeneity by predicting the treatment effect at individual levels. Then I will share the concept and some application examples to maximize the effectiveness of treatment towards a future precision medicine approach.
Bio: Dr. Inoue obtained his MD from the University of Tokyo in 2013 and PhD (Epidemiology) from University of California, Los Angeles in 2021 after clinical training as an endocrinologist. He was appointed Assistant Professor in 2021 at the Graduate School of Medicine, Kyoto University. His research interest is the application of causal inference methods and machine learning in chronic disease epidemiology. From 2016 to 2023, he published 48 first/last authored papers & 28 co-authored papers in medical/epidemiological peer-reviewed journals. For more details, please visit the website: https://endoepi.net/
Location: Seminar Room 505, L5, 207 Bouverie Street or zoom Click here to join the meeting (meeting ID: 86587929600 and password: 821925)